How to mitigate uncertainty in the supply chain?
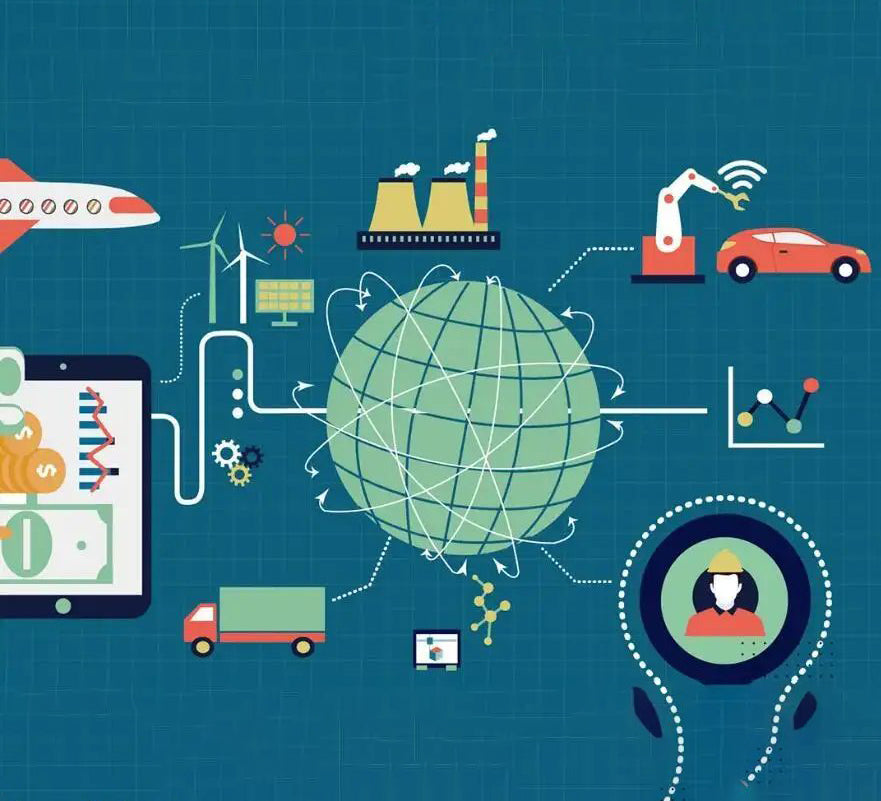
#SupplyChain #Logistics
Uncertainty is an inherent feature of supply chains, and we cannot eradicate it, because the source of demand, each individual consumer thinks differently, and changes from time to time. If you want to drink coffee today and drink milk tea tomorrow, it is difficult to accurately grasp the individual needs.
But interestingly, when we aggregate the demand together, we find that the volatility of the large sample decreases. For example, when ordering afternoon tea, how many people in the team want to drink coffee or milk tea will definitely not be one-sided, and the fluctuation of demand is relatively small.
When we bring together some of the same or similar things, there is a special term in the supply chain called "Pooling" or "Aggregate".
For example, when making forecasts, integrate the same requirements. When calculating the capacity, the same workstation capacity is aggregated and counted. This practice can reduce volatility, and today I will focus on this aspect.
1. Why do pooling?
Let's use an example to further illustrate what's going on with demand volatility. As mentioned above, there is a lot of randomness in consumer buying behavior, which creates volatility. Zhang San opened a coffee shop, and in order to increase the unit price, the shop also sold cakes.
How should he stock up? There are too many purchases, and the cakes are not sold out. When the shelf life is reached, the cakes will be discarded and thrown away. Less purchases, insufficient inventory, and loss of sales.
In order to better stock up, Zhang San counted the sales of cakes in the store in the past 20 days. Zhang San's store is open five days a week, 20 days a month, and the specific sales are as follows.
Days | Sales Quantity | Difference from Mean Sales | Difference in Absolute Value |
1 | 42 | 7 | 7 |
2 | 30 | -5 | 5 |
3 | 24 | -11 | 11 |
4 | 35 | 0 | 0 |
5 | 43 | 8 | 8 |
6 | 38 | 3 | 3 |
7 | 34 | -1 | 1 |
8 | 29 | -6 | 6 |
9 | 44 | 9 | 9 |
10 | 21 | -14 | 14 |
11 | 33 | -2 | 2 |
12 | 42 | 7 | 7 |
13 | 46 | 11 | 11 |
14 | 31 | -4 | 4 |
15 | 37 | 2 | 2 |
16 | 31 | -4 | 4 |
17 | 44 | 9 | 9 |
18 | 36 | 1 | 1 |
19 | 41 | 6 | 6 |
20 | 27 | -8 | 8 |
Average | 35 | 0 | 6 |
We saw a peak of 46 sales and a low of 21, with an average of 35 (rounded). The actual results for sales volume vary widely from the average, but they average zero because of the positive and negative offsets.
We want to take the absolute mean of the difference, this number is also called MeanAbsolutely Deviation (MAD), and its monthly mean is 6.
From a statistical point of view, we should use the standard deviation, which is more rigorous. After a simple calculation in Excel, we can get a series of analysis results about this month's sales.
Max | 46 |
Minimum | 21 |
Average | 35 |
Standard Deviation | 7.1 |
Coefficient of variation | 0.2 |
The Coefficient of Variation (CV) is the standard deviation divided by the mean, and the result is 0.2. From a statistical point of view, this month's sales are very stable.
From a daily perspective, the maximum absolute difference between daily sales and the average value is 14, which is 40% divided by the average value of 35, which is the magnitude of the deviation.
When the deviations of these 20 days are summed up, the average absolute value difference is 6, which is 17% divided by 35, which is obviously much more stable than 40%. This is because the sales volume is high and low, and the statistics are calculated in groups of 20 days. The difference in sales above and below the average is canceling each other out, so the overall view is more stable.
It is difficult for Zhang San to stock up only by looking at the daily sales data, because the daily sales fluctuate greatly, but the monthly demand is relatively stable, which can provide more accurate instructions for stocking.
2. Why Pooling can make random variables more stable?
Next, we will look at why Pooling makes random variables more stable from statistical theory.
The coefficient of variation is a summary statistic that measures dispersion and is often used to compare different items, such as two different needs or products. We can compare their coefficients of variation to see how similar or different their characteristics are.
We've seen before that if there is a one-month stochastic, it's the sum of 20 daily stochastics. So we have a monthly random variable M, and 20 daily random variables Di.
Assuming that Di are independent and all of the same distribution, they belong to the normal distribution. We have a normal distribution with mean µ and standard deviation σ.
And if the random variable of monthly sales also obeys a normal distribution, that is, the daily and monthly distributions are the same, then we need to establish a conversion relationship between these two time scales.
Daily monthly mean µ20 * µ standard deviation σ√20 * σ
First look at the average, because the number of working days per month is 20 days, so a month is equal to 20 days, we can directly multiply the daily average by 20, and wait until the monthly average, which is easier to understand.
The standard deviation is not calculated in this way. According to the formula, the square of the sample size is required, which is √20. The monthly standard deviation is √20 times σ.
The daily coefficient of variation is σ/µ, which is: 1*(σ/µ), and the monthly variation is: (√20*σ)/20*µ=0.22*(σ/µ).
Obviously the monthly coefficient of variation of 0.22 is less than the daily 1. This is a mathematical proof that after the demand is aggregated, the monthly volatility is less than the daily one, which will help us formulate relevant supply chain strategies.
3. Usage scenarios
3.1 Delay strategy
The delay strategy in the supply chain divides the production process of a product into a generalization stage and a differentiation stage. Enterprises produce common components first, and delay the manufacturing process of product differentiation as much as possible.
The differentiated production of the product is not completed until the end user requests the appearance or function of the product. For example, for the soy milk in the breakfast shop, the product in the generalization stage of the product is the original soy milk juice, and the differentiated product is the sweet and salty milk processed on the basis of the original juice.
The demand volatility of a single product is definitely greater than that of all products, so breakfast restaurants should aggregate the demand for all soymilk products to make predictions with higher accuracy than any single product.
We also use this approach when forecasting demand for other commodities, because individual commodity demand will vary, but these differences will cancel each other out, making the overall forecast more accurate.
3.2 Inventory settings
Some fresh food e-commerce companies use the front warehouse model to serve the community within a few kilometers around the warehouse.
The deviation in stocking of a single front warehouse will be relatively high. If we combine the requirements of several front warehouses, use a regional general warehouse to cover the needs of these front warehouses, and distribute from the general warehouse to the front warehouses, we can Optimize overall inventory costs and on-time delivery rates.
The total position gathers the demand of each front position and slows down the volatility.
3.3 Production capacity
When we do production planning, we generally plan the capacity of a production line, for example, three types of products A, B, and C can be produced on this line. I may not be able to determine the specific number of A, B and C production, because the actual situation will always change, sometimes there is a shortage of materials, can not produce A, can only do B or C.So when we plan the capacity for a complete production line, we often aggregate all the capabilities. In the monthly production and sales coordination meeting, we may have difficulty deciding on the specific quantity to be produced, but instead plan the total production capacity of the production line. From the above, we know that doing this will be more accurate and less volatile.
In the actual production process of products, unexpected situations will inevitably occur, some are over-completed, some are under-target, but they can indeed compensate each other to achieve our overall goal.
To sum up, Baichuan Juhai Pooling is a very practical supply chain strategy, which can effectively alleviate various volatility. The aggregated dimensions include time and quantity, which need to be flexibly applied according to the situation.
Leave a comment